Data Quality impacting Supply Chain Analytics?
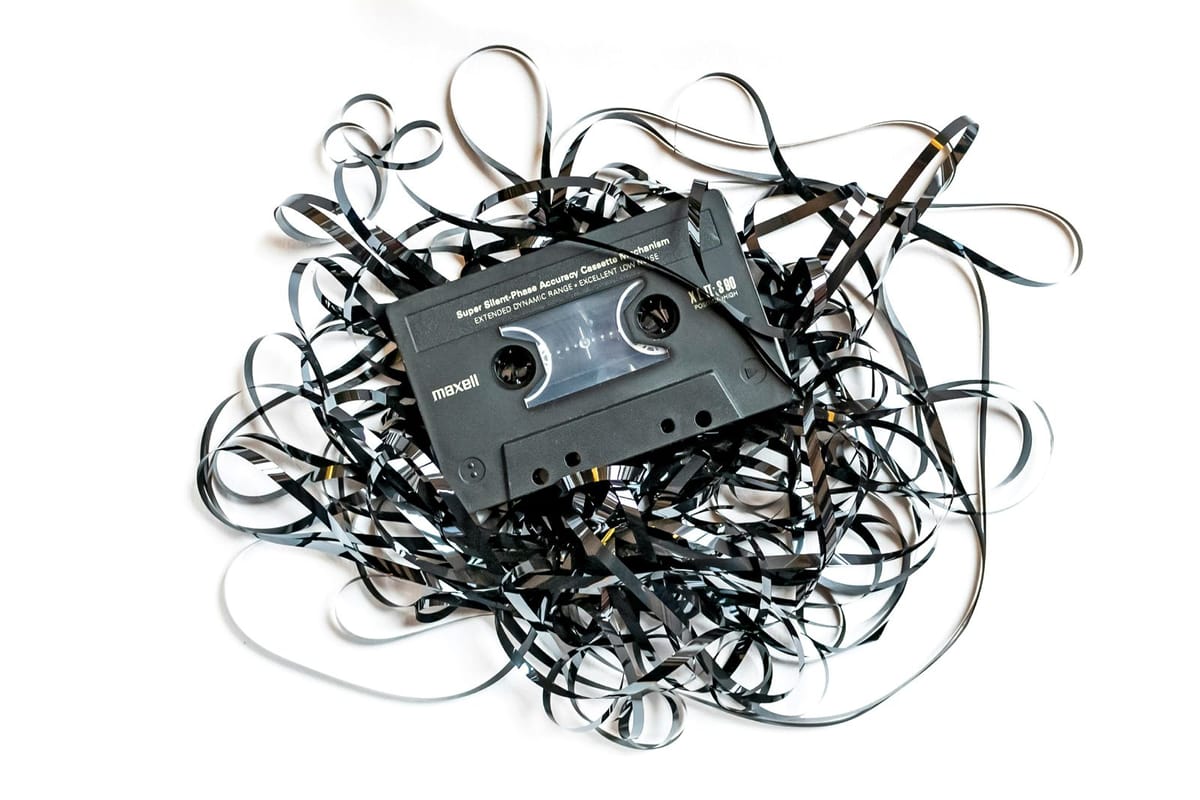
Supply chain analytics is crucial in today's business world, providing organizations with valuable insights into their supply chain operations. By utilizing advanced analytical techniques, businesses can optimize processes, improve efficiency, and mitigate risks across the entire supply chain lifecycle. Supply chain analytics empowers decision-makers to make informed choices, from forecasting demand and managing inventory to optimizing transportation routes and supplier relationships, driving competitive advantage and profitability. Leveraging data-driven insights enables companies to identify cost-saving opportunities, enhance customer satisfaction, and adapt quickly to dynamic market conditions. In today's interconnected global economy, deriving actionable intelligence from supply chain data is essential for staying ahead and delivering superior value to customers.
Various tools are available to assist at different stages of supply chain analytics, aiming to help find solutions. However, many tools fail not because of their quality, but due to the quality of the data they work with. In fact, several sources highlight the well-known "80-20" rule in Data Science, suggesting that 80% of your team's time is spent on collating and cleaning data, while only 20% is dedicated to actual data science or analytics. While these numbers may vary, the underlying message remains true: a significant portion of effort is invested in improving the quality of data.
Here’s a compilation of potential data pitfalls to keep an eye out for -
Data Collection:
- Incomplete Data: A straightforward challenge, but a very common one. One possible solution is to put in Mean/Median values as replacements. This edX article will give you a few more options.
- Inaccurate Data Entry: Human errors during manual data entry can introduce inaccuracies into the dataset.
Statistical adjustments to your data can partially resolve this issue, but a contextual approach may offer a more effective solution. For instance, if vendor A's delivery time is 5 hours for orders containing only item X, but 8 hours for orders with multiple items, leveraging context can provide a superior resolution for missing data.
Data Integration:
- Data Silos occur when data isn't fully integrated from different sources, whether they're your own or competitor/market data. This creates isolated pockets of information, limiting the comprehensive view needed for effective analytics.
- Bringing together data from closed systems owned by different vendors, like ERP systems, inventory management systems, transportation databases, and external sources, poses a challenge due to differences in data structures and connectivity ease.
Data Storage:
- Duplication: Duplicate records in the database can distort analytics results, leading to overcounting and incorrect conclusions.
- Outdated Data: Failure to update or purge outdated data can impact the relevance of analytics insights.
- Latency: Delays in data availability or updates from different sources can impact the timeliness and accuracy of your analytics.
Other Data Transformation Challenges:
- Data Formatting Issues: Inconsistent formatting of data, such as date formats or currency symbols, can lead to misinterpretation.
- Data Enrichment: Enhancing raw data with additional attributes or contextual information from external sources, such as weather data, market trends, or supplier information, can provide valuable insights to help with decisions
There are several clever products out there that solve data quality challenges. Most look at solving these issues holistically, this would mean an organization-wide implementation that could take years. It will be wise to choose your solution keeping time to market in mind.
In conclusion, this is not to discourage you from adopting advanced analytical solutions for your supply chain but more of a guide on what to keep an eye out for.
About Dataring:
At Dataring, we aim to solve the data quality problems using Gen AI. Our approach - Data Alignment, keeps data contextualised with business objectives in mind. This enables us to deliver quick wins solving upstream data pitfalls without the need to reinvent your organization’s data structure. Dataring is also source agnostic, it can connect to your data anywhere.
Our product is continuously evolving. To get the latest head over to our website or reach us on LinkedIn.